Table of Contents
Toggle
Introduction
Generative AI and predictive AI are like AI siblings that have enormous transformative potential for business. These advanced AI models serve different purposes and offer unique advantages to businesses. Generative AI helps businesses generate unique ideas and drive innovation in the form of data. Predictive AI, on the other hand, excels at forecasting future events and trends by analyzing historical data for business. But how do you choose which AI will be the right fit for your business objectives? By going through this article, you can decide which technology is best for your organization.

What is Generative AI?
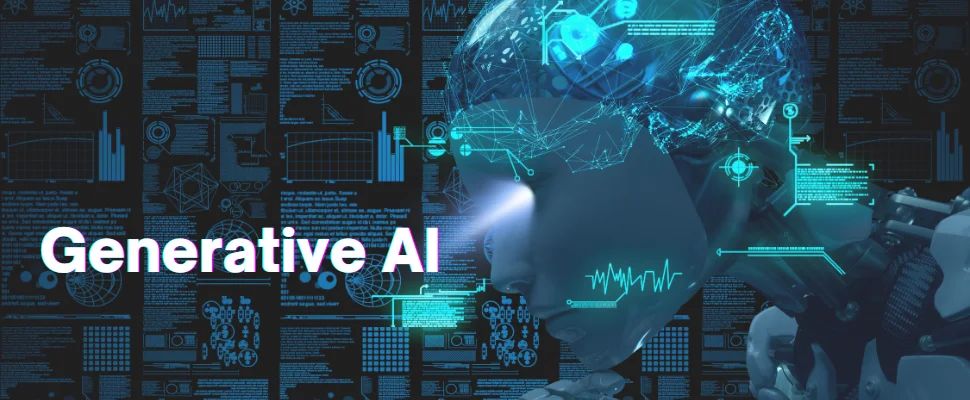
Generative AI is a subset of artificial intelligence that can generate unique outputs that exhibit creativity and originality. It uses generative adversarial networks (GANs), variational autoencoders (VAEs), and autoregressive models to learn patterns and generate human-like content, compose music, and images, and even design virtual worlds. This AI model helps many designers, artists, and content creators explore their ideas and translate them into reality.

What are the Types of Generative AI?
Transformer Models: This model helps natural language processing (NLP) understand sequential data by focusing more on relevant words in long sentences. In the context of generational AI, transformers play a crucial role in generating new content. These models are trained on large datasets to identify the complex patterns in the sequential data.
Generative Adversarial Networks (GANs): Using GANs, generative AI can generate unique, realistic images. It uses two types of neural networks, i.e., a generator and a discriminator, where the generator processes the image from the user’s input and the discriminator takes both real and AI-generated input and then determines which one is fake and which is real. This technology is favorable for businesses with tasks like product design, art, and content creation.
Variational Autoencoders (VAEs): This type of model can analyze the data within its datasets. By using compression and generation, they take high-dimensional input, such as images, into their low-level dimensional space. Once it compresses, VAE generates new data from the compressed space.

Pros of Generative AI
- Best for Automating Monotonous Tasks: Generative AI models can make repetitive tasks easy. Whether it’s crafting concise product descriptions from draft emails, code snippets, marketing content, or layouts, generative AI excels at it. This, in turn, helps save time and enhances productivity across organizations.
- Produces Fresh and Innovative Content: Generative AI can elevate your creativity to the next level. It helps to break the boundaries for designers and artists.
- Personalized Marketing Campaigns: Generative AI helps you tailor content to individual preferences. So, you can use this for personalized marketing campaigns to create a compelling product description. Adding a personal touch to your campaigns can enhance customer engagement and satisfaction. As a result, it can boost performance in various contexts.

Cons of Generative AI
Undeniably, generative AI is beneficial in so many ways, but it comes with some flaws as well. The following are some of the risks of generative AI that businesses should be careful about:
- Generative AI is Unpredictable: Sometimes Generative AI lacks transparency in their responses. Since the AI model is programmed to generate the output independently, some of the content it produces may be false or incorrect. Therefore, this unpredictable behavior of generative AI makes it hard to work, especially when accountability is crucial.
- Biased Responses: Businesses need to take proactive measures to detect and monitor biased responses from generative AI. This AI model can learn the basic data and produce content in such a way. Thus, businesses must evaluate the AI-generated content.
- Lacks Authenticity: Generative AI models are just in the first phase of development, due to which they produce content that is not authentic and contains inappropriate responses. While using it for use cases, businesses need to do research on it and only map with the AI-generated content, then only share the information.
- Privacy and Security Concerns: Generative AI models can reproduce private and sensitive content from their training; thus, businesses need to safeguard their individual security and sensitive information.
- Copyright Issue: Generative AI models are also trained on copyrighted data. If so, this led to the rise of ethical or ownership concerns. Hence, businesses should include legal standards and guidelines to avoid legal conflicts.

Companies that use Generative AI
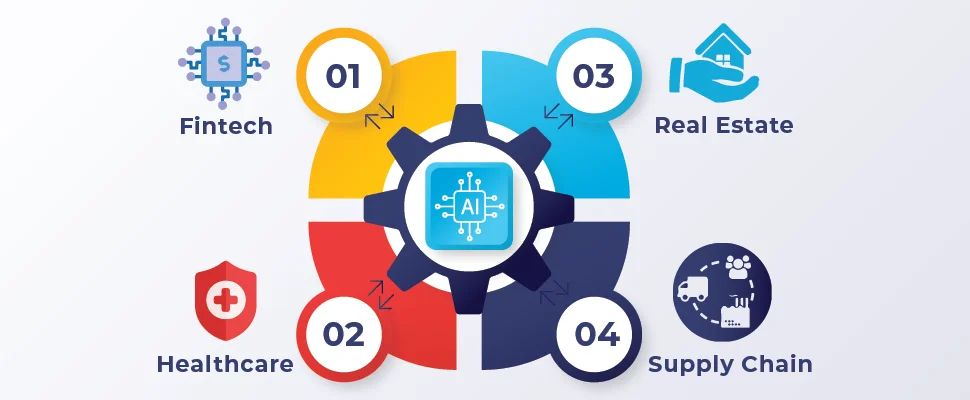
Generative AI can do so much more than just creating content. Automation processes can provide help to various industries.
Fintech: Generative AI is a great tool for banking as it can quickly analyze and detect fraudulent transactions. It can also predict the creditworthiness of an entity or an individual by going through their credit history and employment history. Besides, it is also a handy tool for evaluating strategies such as loans and insurance policies and finding out the future risks.
Healthcare: Generative AI can analyze unstructured healthcare datasets and help in advancing diagnostics, treatment personalization, and pharmaceutical research. It scrutinizes X-rays, CT scans, and MRIs and then detects diseases like cancer, heart disease, and neurological disorders. Using natural language processing (NLP) and electronic health records (EHRs), provides physicians with accurate diagnoses and treatment decisions.
Real Estate: Generative cans will be favorable for real estate agents by helping them accurately determine the value of a property in seconds. And they don’t have to worry about property search and pricing optimization. Generative AI can carry out this task with ease. First, it can search the history and preferences of buyers and then craft personalized property recommendations. Secondly, it takes market trends, demand, and competition into consideration and makes an exact prediction of the rent amount.
Supply Chain: Generative AI can provide a lot of benefits to supply chains. By generating accurate demand forecasts, identifying risk assessments, analyzing demand patterns, and minimizing holding costs, not to mention that its algorithms can optimize the best short routes, which reduces transportation and fuel costs. This, as a result, can help enhance on-time delivery performance.

What is Predictive AI?
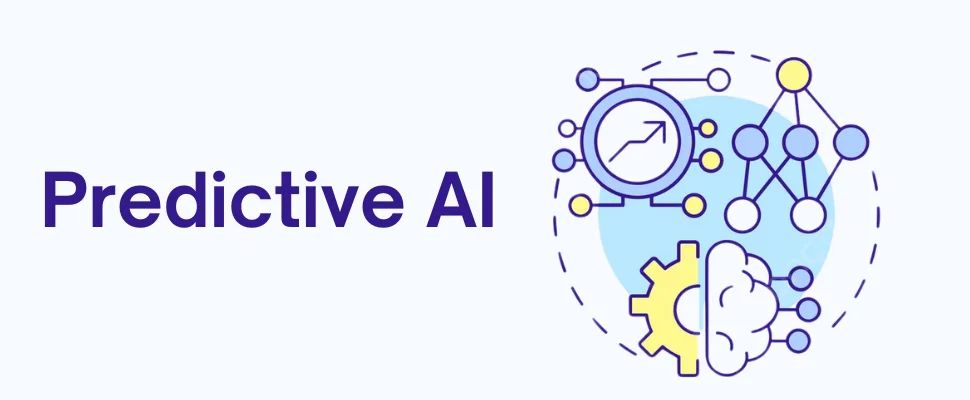
Predictive AI is also another subset of AI; it uses static algorithms and machine learning techniques to analyze existing data or historical data patterns and predict future events or trends for your business. With the predictive AI model, you don’t have to worry about making informed predictions.

Pros of Predictive AI?
- Pinpoint Customer Behavior: Predictive AI is beneficial for businesses to identify customer behavior and make data-driven insights to better understand customers. These analyses help businesses identify their hidden trends and enhance their performance. By accurately identifying the hidden trends in the customer, businesses can increase the likelihood of customers being interested in selling the product.
- Enhance Customer Experience: Predictive AI models are remarkable for predicting future outcomes. By leveraging it, businesses can improve their operations, plan precisely for future demands, and create the best customer experience. Having predictive AI tools can help you determine which types of stocks get quickly out of stock and when they should be restocked, and at the same time, it prevents businesses from overloading with low-selling items. This way, businesses can give the utmost significance to their customers.
- Make Informed Decision: Businesses don’t need to spend their time doing guesswork; using predictive AI, they can make informed decisions. It can analyze vast amounts of data and find out exactly which activities or behaviors may predict positive outcomes. It also predicts the customer’s lifetime value (LTV) based on previous and current data.

Cons of Predictive AI
- Heavily Relies on Trained Data: A predictive AI model relies on the data or program that it is trained on. Therefore, it cannot verify and check the quality of the data. If the given input is not accurate and lacks authenticity, then this predictive model will produce content that is of poor quality and won’t be useful. Hence, business owners can use an automated tool like Pecan to take care of inaccurate and biased data.
- Data Security Concerns: According to the survey, 30% of professionals are concerned about data privacy. Finding the best way to ensure the security of customer data and leverage AI tools can be tricky. Therefore, it is difficult for organizations to protect data privacy with artificial intelligence.
- Steep Expenditure: To carry out predictive analytics, businesses must consider data collection, cleaning, analysis, and more. For this, one must have specialized skills to operate at each stage. This will increase the cost of hiring experts for the team and the maintenance of this AI model.

Real-life use cases of Predictive AI
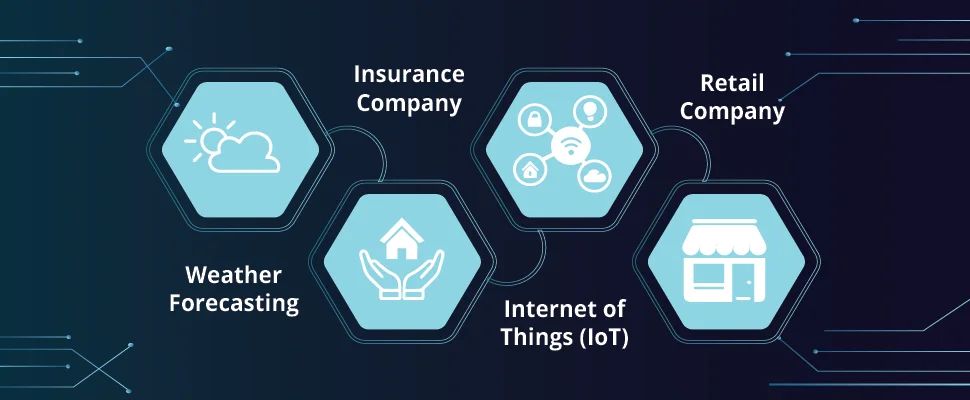
Weather Forecasting: With predictive AI, accurate prediction has become possible. It gathers the existing data available and forecasts when it will rain, how sunny it will be today when a hurricane will occur, whether there will be strong winds, and more. This helps many concerned authorities prepare in advance to fight against the losses and damages.
Insurance Company: Predictive AI can help cut out the extra expenses, as analyzing the risks beforehand could do severe damage. For human counterparts, carrying them out manually would take way longer, but predictive AI effectively helps to resolve unsettled claims on time. It also helps the company to prevent major risks in the future and generate better revenue and estimates.
Internet of Things (IoT): It offers a plethora of advantages, like the ability to analyze real-time data from various IoT devices and predict when equipment is likely to fail. Secondly, it is of great use for monitoring patients’ health using various sensors and other IoT devices for improved care. Also, it can predict peak demand times and implement adequate energy consumption for sensors and various IoT devices. Finally, it can be used to promote increased security.
Retail Company: Predictive AI can offer additional perks to retail companies by analyzing customer trends and sales forecasts based on the insights collected based on data. With accurate insights, companies can easily read customer buying patterns and improve their marketing strategies. Not only that, it considers various parameters for the company to grow and meet customer demands efficiently.
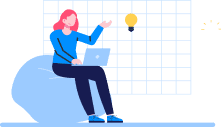
Don't miss out on your chance to work with the best
apply for top global job opportunities today!

Key Differences Between Generative AI and Predictive AI
Generative AI | Predictive AI |
---|---|
Used for creating new information about any content related to marketing, copywriting, or promotional content. | Used to make predictions or forecasts using existing data. |
It can generate text and images. | It can forecast, classify, and perform regression. |
It depends on diverse and comprehensive data. | It makes predictions based on the customer’s historical data or by analyzing the previous data. |
It uses complex algorithms and deep learning, due to which it can produce human-like content. | It depends on statistical algorithms and machine learning to make accurate predictions. |
Trained for more complex and resource-intensive tasks. | In comparison to generative AI, this model requires less complex training. |
This type of AI model learns from patterns and relational data. | This model learns from analyzing historical data to make predictions. |
It can produce new, original content or data. | It can analyze existing patterns of customers or any data and find out the outcomes. |
Lacks authenticity in generating responses sometimes and can’t be specific in output. | It is limited to existing patterns, and sometimes it misses out on novel scenarios. |
It helps you take your creativity to new heights by generating high-quality content. | It doesn’t have the element of content creation. |

What are the benefits of Generative AI in the Telecom Industry?
Generative AI can offer a plethora of benefits to the telecommunications industry. The following are some of the perks of using generative AI in telecom:
- Personalized Content: Generative AI can mirror user behavior and then predict how consumers respond to new services, pricing models, or network changes. Thus, telecom can leverage its generative AI to create personalized content for its customers. Also, using chatbots, they can automatically solve queries and resolve any customer issues. In addition, it can help them provide recommendations for the highest customer satisfaction and turn them into loyal customers.
- Voice and Speech Synthesis: By using synthetic voices, the telecom industry can improve the user experience by providing more natural and diverse voice options. Generative AI models can stimulate the normal behavior of network components and make precise predictions on network performance. If it encounters unusual behavior, then it can take automated measures to prevent the threats.
- Meticulous Planning and Optimization: Generative AI can handle complex telecom networks by strategically planning and optimizing their network. Its machine learning model is capable enough to imitate the network’s behavior and forecast the user’s patterns. Therefore, it can improve the performance and efficiency of telecom network systems.
- Content Generation: The telecom industry needs to generate marketing content, advertisements, and informative articles. Generative AI can analyze user preferences, relevant data, and trends, which can help them come up with innovative ideas and solutions quickly.
- Data Security: Telecom companies work on large volumes of data for testing, training, and research purposes. Generative AI in this regard can offer a great deal of help by mimicking real-life data and helping them test and develop solutions to existing problems. It helps them detect new fraud scenarios and patterns and stay ahead of emerging threats.

Five Cutting-Edge AI Trends to look for in 2024
- Improvements in Multimodal AI models: In 2024, we are going to witness huge improvements in multimodal AI models with advanced language models like Llama 2, GPT4, and Mistral. A new model such as LLava and GPT4-V can create text, images, and audio. By using an advanced algorithm, AI can mix and generate content intuitively.
- Kubernetes a Preferred Hosting Platform: Since tech giants like Hugging Face, OpenAI, and Google use Kubernetes to host their AI modules. There are high chance that Kubernetes will be the trend in 2024. It supports tools such as Ray Serve, vLLM, and Text Generation Inference. Therefore, users can efficiently manage the lifespan of AI models using more sophisticated Kubernetes-based frameworks.
- Alternative to AI Hosting: With the rising popularity of Mistral’s Mixtral-8x7B, Falcon 180B, and Meta’s Llama 2 70B, AI models like GPT 3.5, Claude 2, and Jurassic-2 will be surpassed. And these new AI models can provide better options for different ways of hosting.
- Revolutionized Generative AI: With the use of multimodal AI and advanced algorithms, as well as tools like LlamaIndex and LangChain. It can reshape the way generative AI works. A self-learning system can examine various forms of data to make well-informed conclusions and enhance consumer experiences across several industries.
- Small language models (SLMs): SLMs like Microsoft’s PHI-2 and Mistral 7B can provide high-quality sources like large language models (LLMs). These models require less storage and memory consumption because they can run on low-end hardware. Therefore, the SLM model will be more widely used in 2024.

Generative AI vs. Predictive AI: Which AI tool is beneficial for your business in 2024?
Predictive AI is a powerful tool for businesses to identify trends and help decide the next step. In contrast, generative AI will unlock your creativity and help you explore your imagination. Generative AI uses advanced algorithms and deep learning methods, whereas predictive AI relies on machine learning and statistical algorithms to analyze data and predict future occurrences.
Therefore, generative AI would be great for industries that are into music, fintech, art, supply chains, healthcare, and fashion. Predictive AI, on the other hand, is limited to prediction data and is therefore used in weather forecasting, retail marketing, IoT, and insurance. Generative AI and predictive AI are new levels of technology that have a high potential for development and are widely used in different domains today.
Both technologies are highly versatile and effective. While generative AI is popular for producing innovative content, predictive AI is highly sought-after for its accurate forecasting abilities. By having a solid grasp of these AI models, you can leverage the full potential of these AI models in business operations and use them to improve them.
Take control of your career and land your dream job
sign up with us now and start applying for the best opportunities!
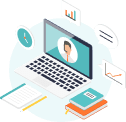
FAQs
Yes, absolutely. By learning predictive AI, you will have excellent career opportunities in business management, data analysis, and many other domains. If you’re interested in pursuing a career in AI, then you must have solid knowledge and expertise in predictive AI.
Generative AI does not perform predictive analyses as it lacks the core functionality for predictive analytics. It mainly generates new content and does not use historical data to make decisions. Therefore, it is not possible to use genetic AI for predictive analyses.
Skills that you must have include expertise in artificial intelligence (AI), data mining, machine learning, statistical modeling, decision trees, regression analysis, and data science technologies. These skills will help you become good at predictive analytics.
Businesses and organizations are not sure and perplexed about their business operations. Predictive AI helps them overcome uncertainty by analyzing market trends, customer behavior, pricing strategies, and more. In addition, it also provides data through which businesses can make well-informed decisions.