Table of Contents
Toggle
Introduction
Conversational AI and generative AI are the two subsets of AI that serve different purposes and goals. While generative AI is popular for generating content, images, and audio, conversational AI is used for creating human-like conversations and context-aware responses. Both AI technologies are phenomenal in their respective domains and have greatly contributed to the advancement of AI. In this blog, we will find out what the difference is between conversational AI and generative AI.

Generative AI vs. Conversational AI: Overview
Generative AI is a creative hub that analyzes patterns using extensive data and existing examples and creates innovative output that even surpasses the human imagination. It is a beneficial tool for a professional who is in the marketing, artistic, or creative fields. Behind the success of generative AI, generative adversarial networks (GANs) play a significant role. This adversarial process leads to the generation of highly realistic content by using two components, i.e., a generator and a discriminator. These components operate effectively by teaching each other to produce better results.
While conversation AI, on the other hand, helps to bridge the gap between AI and humans, it relies on natural language understanding (NLU) and natural language generation (NLG) to mimic human-like conversation. Its ability to understand intent and process the necessary information allows it to generate the right response to customers’ queries instantly.

Conversational AI vs. Generative AI: Key Features
Conversational AI: Its prime focus is to process the natural language conversations between humans and AI by using Natural Language Understanding (NLU) and Natural Language Generation (NLG) for smooth interaction. The following are several key features that enhance conversational abilities:
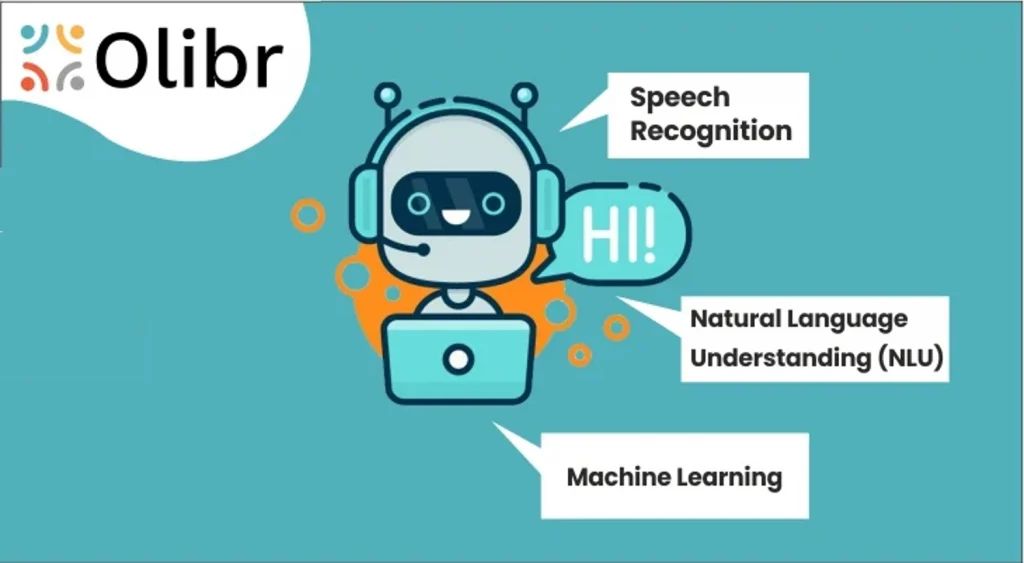
- Speech Recognition: Conversational AI has automatic speech recognition to convert speech into textual form by filtering background noise and disturbances. The use of advanced algorithms enables them to understand human voice input and process it in the form of speech or voice commands. With every interaction, the AI engine becomes smarter, which provides enhanced user experiences and fosters business growth.
- Natural Language Understanding (NLU): Sophisticated NLU encompasses lexical analysis, semantic analysis, sentiment analysis, and output transformation. This further helps in analyzing raw user input and then generating relevant responses as per the user’s inquiries or commands.
- Machine Learning: At its core, machine learning (ML) helps conversational learners comprehend complex algorithms to carry out coherent and context-aware conversations. Continuously learning and finding out patterns from large data sets, conversational AI understanding, and response mechanisms in a natural, human-like manner. Some of the examples are ML-powered image recognition and even self-driving cars.
Generative AI: It is mainly used for generating unique content using machine learning algorithms and leveraging deep learning techniques. It also relies on neural networks to generate realistic and creative outputs in text, image, and audio form. The following are the key features of generative AI that are extremely popular today:
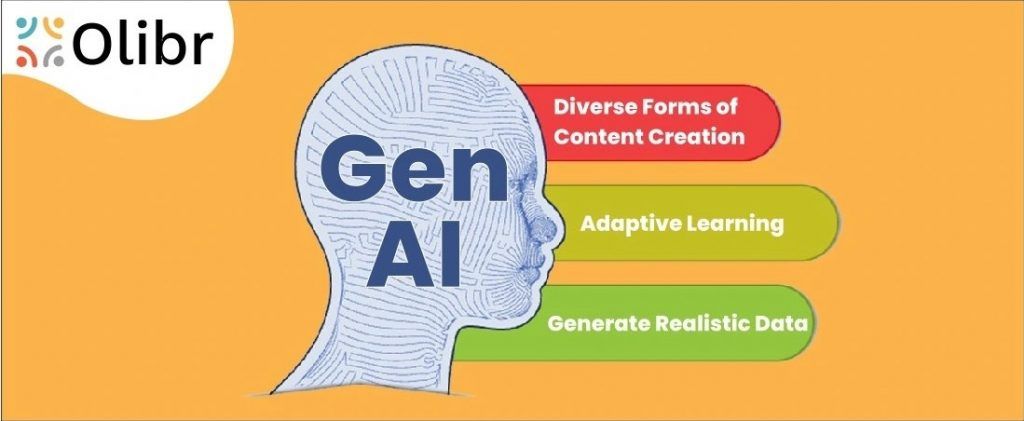
- Diverse Forms of Content Creation: Gen AI analyzes patterns and structures in the training data and then creates unique content based on the data in the user’s input. Thus, its ability to create novel content and generate creative images, music, audio, and video makes it a valuable tool for everyone.
- Adaptive Learning: It can quickly learn and adapt new data based on user feedback. This nature of Gen AI helps it improve its performance over time and generate better results that align well with user preferences and objectives. Some examples of Gen AI are dynamic learning styles, materials, and techniques to match every student’s requirement and improve learning experiences.
- Generate Realistic Data: It uses a variational autoencoder (VAE) model to understand complex data and trains on extensive datasets to better understand the underlying patterns and generate fresh samples and image restoration.
By going through these key features, we can see that conversational AI is different from general AI. It focuses more on delivering a response like a human and answering commands and speech by using natural language understanding (NLU) and natural language generation (NLG). Whereas Gen AI is quite advanced and has immense potential to enhance productivity in diverse domains.

Difference Between Generative AI and Conversational AI
Conversational AI | Generative AI |
---|---|
It needs consistent data quality for measuring the accuracy and effectiveness of the system. | It can effectively handle the various user inputs, but sometimes it generates biased and less reliable outputs, which need monitoring. |
It prioritizes prompt user interactions and adapts well to increasing user interactions, ensuring consistent performance. | It takes time to process intricate content generation, but it scales effectively with data and refines content over time. |
Sometimes AI cannot process highly technical queries, and when asked out-of-context, it struggles to provide the relevant responses. | It can produce new and fresh outputs, but sometimes it lacks relevant context. |
It can comprehend and respond to complex user inquiries, making it a perfect fit for customer service. | It can produce innovative and unique outputs. |
Focus on dialogue management between humans and AI by using natural language processing (NLU). | Used for generating unique and novel content by using the deep learning technique and based on trained large datasets. Its neural networks can quickly fetch the relevant data that users are looking for. |
It takes user feedback and uses iterative model enhancements to provide better experiences. | It sometimes makes changes to the output based on scrutinized data but lacks direct user feedback. |
Take the human’s natural language as input and make a relevant response to it. | It relies on learned patterns and then processes various kinds of data to generate fresh content. |
It relies on high-quality and diverse conversational data to respond correctly to human commands. | It depends on its extensive data types to harness the best content generation mechanisms. |
Adapts to a response based on real-time interactions with humans, answers questions, and provides feedback. | It can provide content using its training data and pattern for content generation. |

Real-life Examples of Conversational AI
With instant prompts and personified responses, conversational AI is an integral tool for businesses to provide the utmost customer satisfaction and drive growth. The following are a few examples of contextual AI:
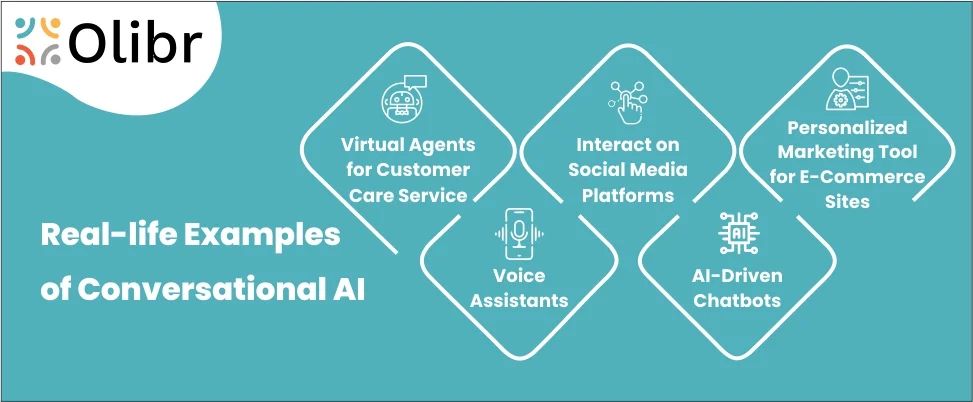
- Virtual Agents for Customer Care Service: Conversational AI excels at handling common questions by using a repetitive and predictable pattern. It can be the perfect tool for customer care support.
- Interact on Social Media Platforms: Businesses these days are leveraging social media platforms to interact with their customers, respond to their inquiries about products, make purchases, and provide recommendations.
- Personalized Marketing Tool for E-Commerce Sites: It can analyze user interactions and provide personalized product recommendations and promotional offers to improve the shopping experience.
- Voice Assistants: Alexa, Google Assistant, and Siri are conversational AI that is used for setting reminders, playing music, providing weather updates, and more
- AI-Driven Chatbots: These chatbots are perfect for handling customer queries, FAQs, and finding the right resolution for complex issues. It can operate 24/7 to solve customer concerns independently, without any human interference.
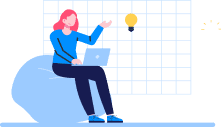
Don't miss out on your chance to work with the best
apply for top global job opportunities today!
Real-life Examples of Generative AI
Generative AI can help businesses improve sales, enhance customer service, and handle automated tasks. The following are examples of general AI:
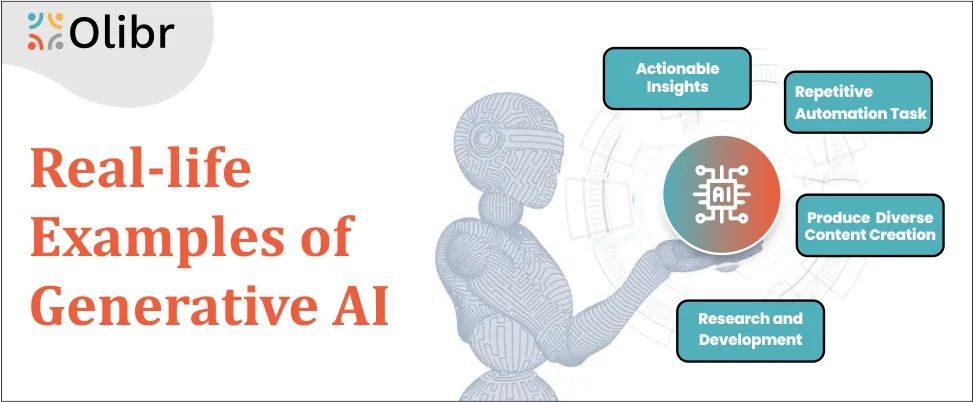
- Actionable Insights: Generative AI can analyze large unstructured data sets and extract actionable insights through summarizations. This is extremely useful for the telecom industry, especially for those who run e-commerce sites.
- Repetitive Automation Task: The healthcare sector, hospitality, and retail industries can use general AI for repetitive tasks like localizing marketing content in multiple languages, replying to requests for proposals, and checking customer contracts for compliance.
- Produce Diverse Content Creation: Gen AI modules like ChatGPT, Google Bard, DALL-E, Midjourney, Musenet, and Runway are beneficial tools for creative industries to accelerate their ideas and go beyond their imagination.
- Research and Development: Generative AI can find a novel solution by going through the existing data. This makes it best for material science development and research.
Generative AI vs. Conversational AI: Which One Should I Choose?
Choosing between these AI technologies depends on your objectives and end goals. Conversational AI can mirror human responses and correctly reply to any command. This is the best fit for user interaction. Meanwhile, Gen AI is more used in generating new content for diverse domains. One of the most popular general AI tools widely used these days is ChatGPT, which is the perfect mix of conversational and generative AI.
Conversational AI and generative AI are two important components of AI, each playing a pivotal role in advancing technology. While conversational AI helps us interact better with AI systems, generative AI allows us to take our creativity to unprecedented heights. The intersection of these technologies opens new possibilities, such as more advanced and creative chatbots in the upcoming year.
Take control of your career and land your dream job
sign up with us now and start applying for the best opportunities!
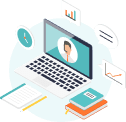
FAQs
Businesses use conversational AI to improve customer service through AI tools like chatbots and virtual assistants. Generative AI is mostly used for content creation, design processes, and product development.
Some of the risks of these AI models are that their outputs are sometimes biased and incomplete. Businesses must monitor and verify the AI-generated results.
Yes. When these AI technologies combine, this combo pack can better understand context and engage users on a deeper level, resulting in a positive impact on the user experience.
Conversational AI and Gen AI are here to stay for the long run, and the future looks promising. With the advancements in deep learning and neural networks, both AI technologies are more sophisticated and integrated into diverse domains. Since businesses are becoming more aware of its potential, there will be a high demand for AI-driven solutions.